There’s more AI news out there than anyone can possibly keep up with. But you can stay tolerably up to date on the most interesting developments with this column, which collects AI and machine learning advancements from around the world and explains why they might be important to tech, startups or civilization.
Before we get to the research in this edition, however, here’s a study from the ITIF trade group evaluating the relative positions of the U.S., EU and China in the AI “race.” I put race in quotes because no one knows where we’re going or how long the track is — though it’s still worth checking who’s in front every once in a while.
The answer this year is the U.S., which is ahead largely due to private investment from large tech firms and venture capital. China is catching up in terms of money and published papers but still lags far behind and takes a hit for relying on U.S. silicon and infrastructure.
The EU is operating at a smaller scale, and making smaller gains, especially in the area of AI-based startup funding. Part of that is no doubt the inflated valuations of U.S. companies, but the trend is clear — and perhaps an opportunity for investors is as well, who might see this as an opportunity to get in on some high-quality startups without needing quite so much capital.
The full report (PDF) goes into much more detail, of course, if you’re interested in a more granular breakdown of these numbers.
If the authors had known about this new Amazon-funded AI research center at USC they probably would have pointed at it as a good example of the type of partnership that helps keep U.S. production of AI scholars up.
A touch of class
On the farthest possible end from monetization and practical application, we have two interesting uses of machine learning in fields where human expertise is valued in different ways.
At Switzerland’s EPFL, some music-minded boffins at the Digital and Cognitive Musicology lab were investigating the shift in the use of modes in classical music over the ages — major, minor, other or none at all. In an effort to objectively categorize thousands of pieces from hundreds of years and composers, they created an unsupervised machine learning system to listen to and categorize the pieces according to mode. (Some of the data and methods are available on GitHub.)
“We already knew that in the Renaissance, for example, there were more than two modes. But for periods following the Classical era, the distinction between the modes blurs together. We wanted to see if we could nail down these differences more concretely,” he explained in a university news release.
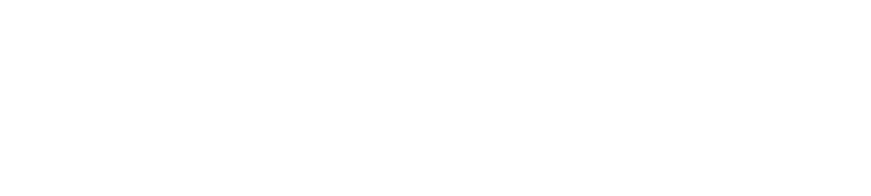