The 2018 World Cup, which ended Sunday, was an almost unequivocal success. The soccer was scintillating, there was little trouble away from the pitch, and the controversial VAR replay system largely delivered as promised.
As with any World Cup, numerous players rose to the occasion, with a breakout performance from France’s Kylian Mbappe among the highlights.
Among those that didn’t perform, however, were big financial institutions. Before the tournament, banks including Goldman Sachs, ING, and UBS all took a stab at a forecasting what would happen, generally by applying techniques traditionally used in financial analysis and economic modeling to soccer.
All but one of them — Nomura — got the tournament wrong.
Of the five major banks to forecast a winner before the tournament, two chose Spain, one chose Germany, and one chose Brazil. Goldman Sachs even changed its modeling several times during the tournament and still managed to get it wrong. Only the Japanese lender Nomura managed to correctly predict that France would leave Russia as the world champion.
So what went wrong? To understand where lenders slipped up, it is first important to look at exactly how they tried to make their forecasts and what they got wrong. Each bank is different, so we’ll look at some of them individually.
Goldman Sachs
Goldman Sachs used perhaps the most interesting form of modeling, employing machine learning to run 200,000 models, mining data on team and player attributes, to help forecast specific match scores. The bank then simulated 1 million variations of the tournament to calculate the probability of advancement for each squad.
“We are drawn to machine learning models because they can sift through a large number of possible explanatory variables to produce more accurate forecasts than conventional alternatives,” a group of strategists from Goldman’s international research team wrote in a client note before the tournament.
“We capture the stochastic nature of the tournament carefully using state-of-the-art statistical methods and we consider a lot of information in doing so,” they added.
“England meets Germany in the quarters, where Germany wins; and Germany meets Brazil in the final, and Brazil prevails,” the bank said in its first forecasts, as evidenced by the chart below:
In reality, Germany was eliminated at the group stage, England made it all the way to the semifinals, and Brazil lost to Belgium in the quarterfinals. Just 11 of the teams Goldman expected to make the last 16 progressed from the group stage, only half of the predicted quarterfinalists made it that far, and just one predicted semifinalist got that far.
Goldman even forecast that the eventual runner-up, Croatia, would be eliminated from the tournament at the group stage, exiting at the expense of Iceland.
After the group stage, Goldman updated its predictions but continued to miss the mark, with its final forecast — delivered ahead of the semifinals matches, England versus Croatia and Belgium versus France — saying we’d see a final between England and Belgium. The final, of course, was played between Croatia and France.
Goldman did caveat its failures before the tournament, saying “forecasts remain highly uncertain, even with the fanciest statistical techniques, simply because football is quite an unpredictable game.”
UBS
The Swiss giant UBS’ modeling was not as extensive as Goldman’s, with the bank running just 10,000 simulations of the tournament.
UBS created its predictions by inputting a series of variables, including the ELO ranking of teams — which is an objective measure of how good they are — into a statistical model and then running that model through a so-called Monte Carlo simulation. Here’s the bank’s explanation from before the tournament:
“To account for the many different roads to the final, we conduct what statisticians call a Monte Carlo simulation. What might sound fancy to some readers is in practice quite simple: Instead of mapping out all the different constellations, we draw a large number of random variables and use these to bring in a random component to our calculations and to simulate the championship. A better team is still more likely to win than its lower ranked opponent, but this is sport, and upsets DO happen. After we’ve repeated this again and again, we end up with the results of 10,000 virtual tournaments. We then simply count how many times each team won, made it to the semis, or was already eliminated in the group stage.”
Rather than trying to predict an exact bracket as Goldman Sachs did, UBS gave each team a probability of progressing through each round of the tournament and a probability of winning it. In this model, UBS backed Germany to win the tournament. Germany, of course, went out in the group stage— an outcome UBS said had a less-than-10% chance of happening.
France was just the fifth favorite in UBS’ model. But even more surprising is that Croatia was given a less-than-1% chance of making it to the final and only a 24% chance of making it out of its group.
Staff at UBS Wealth Management, which compiled the forecasts, acknowledged getting it wrong but pointed to getting about two-thirds of its group-stage predictions correct.
“The big surprise for us was runner-up Croatia,” the firm’s chief investment officer, Mark Haefele, said in an email to clients seen by Business Insider. Croatia won the group containing Argentina and went on to beat Denmark, Russia, and England.
Haefele also said investors could learn from the results, adding that they should always “be sure not to confuse probabilities for certainties — everything in investing (like in football) is only ever likely, probable, or possible, and never certain.”
“Even the best looking investments can underperform,” he said.
ING and Nomura
ING took a particularly novel — and ruthlessly capitalistic — approach to its forecasts, using the overall value of each team in the tournament to predict the winner. ING plumped for Spain, whose squad had a combined value of $1.187 billion. France came second in this forecast, with a value just under that at $1.183 billion.
Nomura, the only bank to pick the right winner, put forward this methodology before the tournament:
“Being analysts, we have to apply some rigor to our World Cup predictions, so we’ve decided to apply portfolio theory and the efficient markets hypothesis to the World Cup. We look at the value of players in each team, the momentum of team performance and historical performance to arrive at three portfolios of teams to watch.”
Why did they get things wrong?
Quite simply, most banks were wrong because of how unpredictable this year’s World Cup was. Germany’s exit, for example, was characterized by UBS’ Haefele as “probably one of the biggest surprises in the more recent history of the tournament.” Germany had not been eliminated in the first round of the competition since 1938.
Away from Germany’s elimination, the penalty-shootout victory of Russia against Spain, England’s progress to the semifinals, and Argentina’s huge underperformance throughout the tournament were all massive shocks and also flew in the face of the data.
Shocks in the group stage created a lopsided bracket in the second round, where one side of the tournament was stacked with favorites to win the tournament. This helped to exacerbate the discrepancies between bank forecasts and reality.
Ultimately, with an event like the World Cup, relying on statistics is a fool’s game. Soccer is simply too unpredictable to be reduced to a statistical model. Sure, statistics can tell us a lot, but they cannot account for the burning pace of France’s Mbappe, the impeccable game management of Croatia’s Luka Modric, or the once-in-a-generation vision of Belgium’s Kevin De Bruyne.
As Goldman Sachs said before the World Cup: “This is, of course, precisely why the World Cup will be so exciting to watch.”
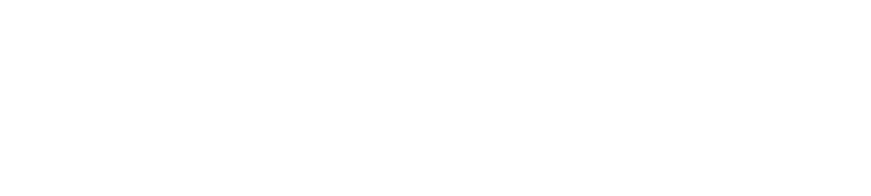