Artificial intelligence and machine-learning technologies have evolved a lot over the past decade and have been useful to many people and businesses, especially in the realm of finance, banking, investment and trading.
In these industries, there are many activities that machines can perform better and faster than humans, such as calculations and financial reporting, as long as the machines are given the complete data.
The AI tools being built by humans today are becoming another level more robust in their ability to predict trends, provide complex analysis, and execute automations faster and cheaper than humans. However, there has not been an AI-powered machine built yet that can trade on its own.
There are many activities that machines can perform better and faster than humans, such as calculations and financial reporting, as long as the machines are given the complete data.
Even if it was possible to train such a system that could replace human judgment, there would still be a margin of error, as well as some things that are only understandable by human beings. Humans are still ultimately responsible for the design of AI-based prediction machines, and progress can only happen with their input.
Data is the backbone of any prediction machine
Building an AI-based prediction machine initially requires an understanding of the problem being solved and the requirements of the user. After that, it’s important to select the machine-learning technique that will be implemented, based on what the machine will do.
There are three techniques: supervised learning (learning from examples), unsupervised learning (learning to identify common patterns), and reinforcement learning (learning based on the concept of gamification).
After the technique is identified, it’s time to implement a machine-learning model. For “time series forecasting” — which involves making predictions about the future — long short-term memory (LSTM) with sequence to sequence (Seq2Seq) models can be used.
LSTM networks are especially suited to making predictions based on a series of data points indexed in time order. Even simple convolutional neural networks, applicable to image and video recognition, or recurrent neural networks, applicable to handwriting and speech recognition, can be used.
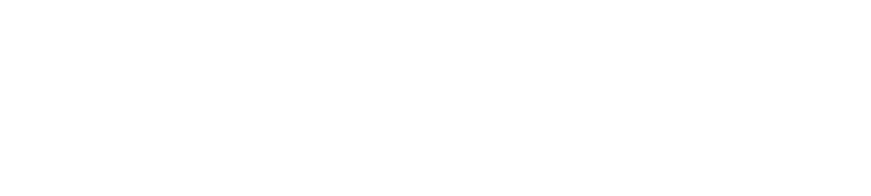